Multi-fidelity Simulations
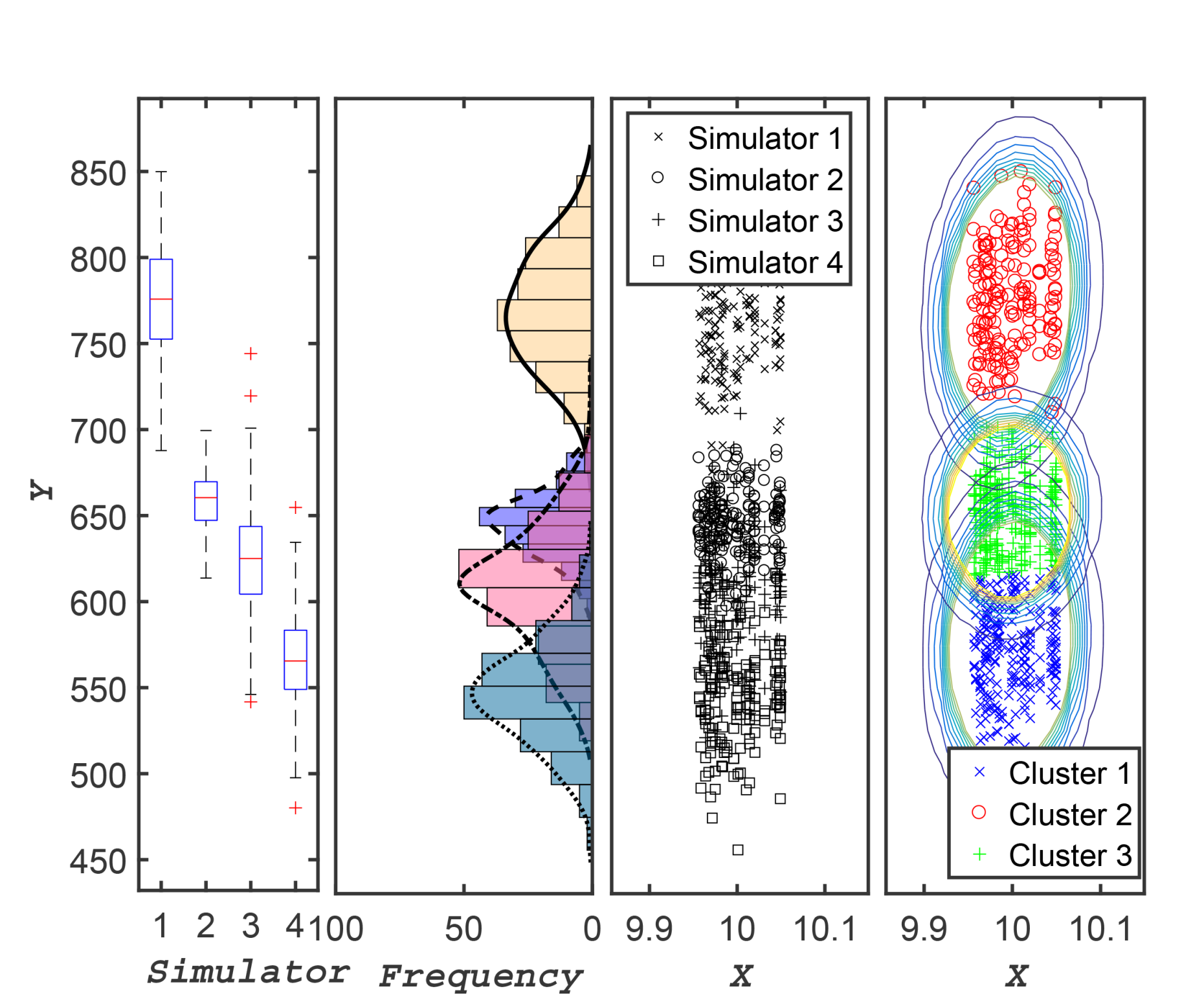
Ensemble aggregation of multi-fidelity simulations & measurements for uncertainty quantification
We propose an ensemble learning approach to aggregate output from multiple simulators and measurements of the same structure. The objective is to investigate how this approach affects the model-form uncertainty, often an overlooked topic in uncertainty quantification. In many instances, stochastic predictions from multiple simulators for the same quantity of interest Yare available. The problem arises when one is unable to prove that one simulator is superior to the others in terms of their predicted output nor establish a clear fidelity-based hierarchy of the simulators. To this end, we propose an ensemble learning approach that rather than treating the predictions of the simulators as competing individual information sources, considers these as part of an ensemble. In the absence of any available measurements D, and based solely on available stochastic output from multiple simulators, the approach consists in first deriving the simulators weights using Variational Bayesian Gaussian mixture clustering (VBGM) and then using the weights to perform a weighted ensemble aggregation on the stochastic output via Bootstrap Aggregation, a.k.a Bagging. The aforementioned weights are derived purely based on an unsupervised learning approach, where apriori information about the performance of any given simulator in a given point in the input space is not available or is highly uncertain. In other words, the derived weights may be inadequate because the method fails to recognize certain simulators are more fitting than others in certain regions of the input space. We tackle this issue by advancing the method, exploiting measurements to update and condition the weights. The first approach presumes that the actual measurements are just another set of "simulators", which are added to the bucket of available output from numerical simulators. VBGM clustering and Bagging is then re-run with the extended space of simulators which now includes the actual set of measurements. This way of introducing measurements to update the weights is necessarily ad-hoc (it has only an intuitive meaning). Alternatively, we explore Bayesian model averaging (BMA) and Bayesian model combination (BMC) which provides a theoretical basis for selecting from an ensemble of combinations.
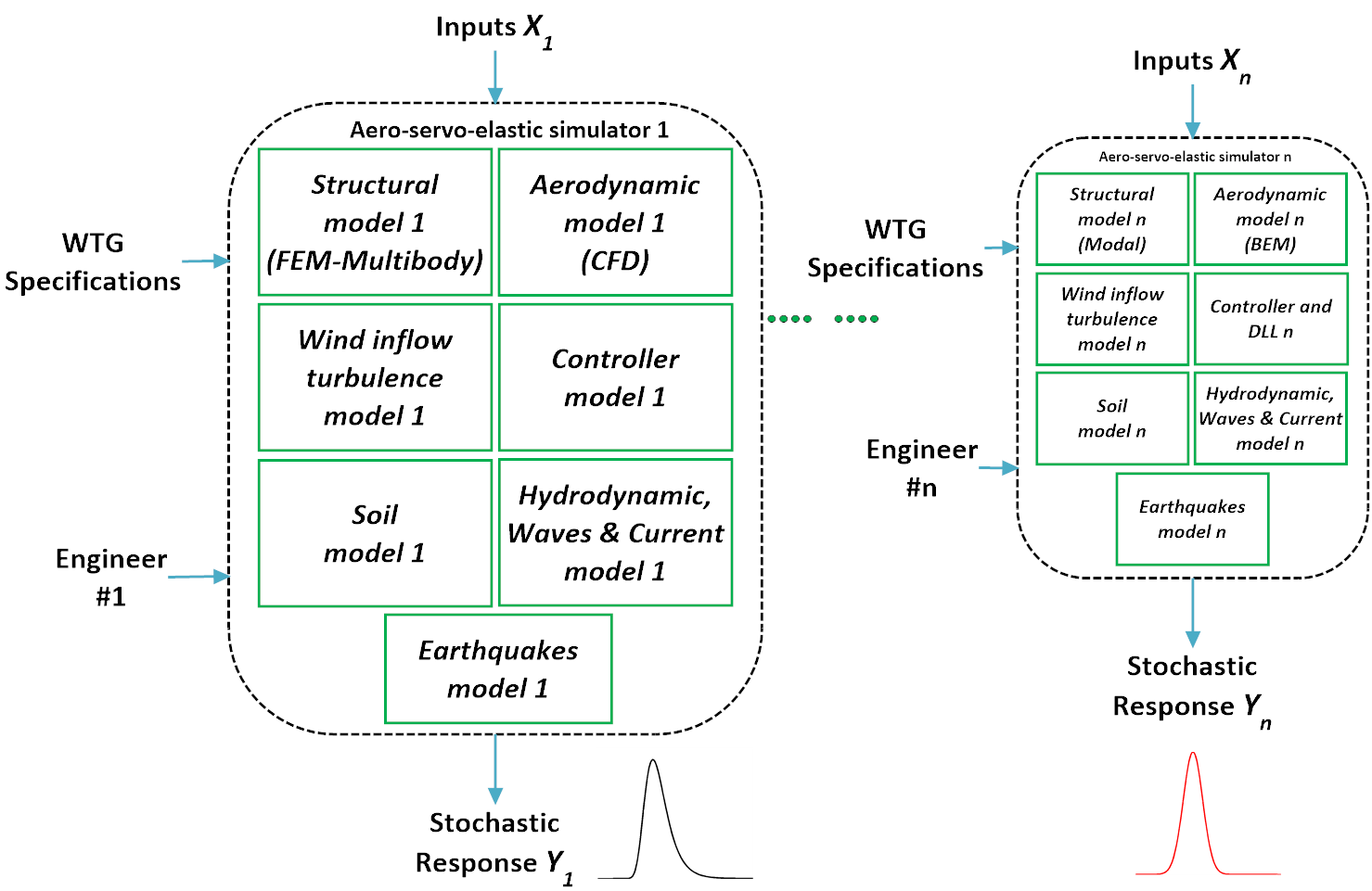